URL: https://www.desy.de/news/news_search/index_eng.html
Breadcrumb Navigation
DESY News: Computer controls plasma accelerator
News
News from the DESY research centre
Computer controls plasma accelerator
A team of researchers has been using machine learning to teach a compact particle accelerator to produce customised beams for a number of different applications. This technique expands the conceivable range of applications for so-called laser-plasma accelerators, innovative compact next-generation accelerators that are currently under development. The scientists led by DESY researcher Sören Jalas are presenting their technique in the journal Physical Review Accelerators and Beams.
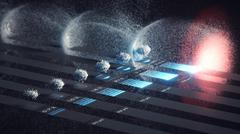
Within a laser-plasma accelerator, a high-intensity laser pulse (depicted in red) generates a plasma wave (depicted in white) in an ionized gas. This wave allows for the acceleration of electron bunches to extremely high energies, spanning a distance of just a few millimeters. Utilizing machine learning, the scientists at LUX can now control the properties of these electron bunches like charge and energy spread (shown in rows here) with high precision. Credit Science Communication Lab for DESY
However, plasma accelerators can be a thousand times smaller than conventional machines. While this has great advantages in terms of building, operating and using them in practical applications, it also makes controlling the beam parameters a challenge. “The acceleration takes place in a very small space, and because of this, the various settings affect each other,” explains Maier. “So if we change one property of the accelerated electron bunch, such as the number of electrons, this can easily affect another property, such as the energy spread of the electrons.”
To prevent this, accelerator physicists must, in principle, gently adjust all of the settings at once to balance the different properties of the electron bunch. However, it is very difficult for human beings to find the right compromise between the many different parameters. In the present case, six different parameters were involved.
“Computers are much more adept at finding their way around such high-dimensional parameter spaces,” says Sören Jalas, lead author of the study. “For our accelerator, we used a method called Bayesian optimisation. Basically, the computer takes control of the accelerator. It tries out a number of configurations and measures the parameters of the electron bunches produced by the accelerator. From these measurements, the computer gradually builds up a kind of map and hence finds tuning curves which show us the optimal way of adjusting the parameters to get the properties of the electron bunch that we need in a given situation.”
In their experimental plasma accelerator LUX, the researchers initially used the technique to adjust the energy and electric charge of the particle bunches. “But it could conceivably be extended to other beam properties, such as emittance,” says Jalas. The method is also to be used in the large plasma accelerator KALDERA, which is currently being built at DESY.
“This use of machine learning brings the practical application of plasma accelerators within reach and at the same time expands the potential uses of these compact machines,” as Wim Leemans, Director of DESY’s Accelerator Division, points out.
Reference:
Tuning Curves for a Laser-Plasma Accelerator; S. Jalas, M. Kirchen, C. Braun, T. Eichner, J. B. Gonzalez, L. Hübner, T. Hülsenbusch, P. Messner, G. Palmer, M. Schnepp, C. Werle, P. Winkler, W. P. Leemans, and A. R. Maier; „Physical Review Accelerators and Beams“, 2023; DOI: 10.1103/PhysRevAccelBeams.26.071302