URL: https://www.desy.de/news/news_search/index_eng.html
Breadcrumb Navigation
DESY News: ChatGPT, run my particle accelerator!
News
News from the DESY research centre
ChatGPT, run my particle accelerator!
Do you sometimes find yourself thinking, ‘I wonder what ChatGPT has to say about this’? It has become common practice to consult artificial intelligence (AI) on a wide range of topics and problems. Scientists at DESY and the University of Hamburg have now asked this question with regard to operating an accelerator and have discovered that ChatGPT can help them to run these machines. They recently published their findings in the journal Science Advances.
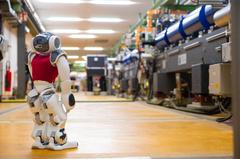
Ein humanoider NA humanoid Nao robot visits the tunnel of the ARES particle accelerator (symbolic photo). Photo: DESY, Jan Kaiserao-Roboter besucht den Tunnel des ARES-Teilchenbeschleunigers. Symbolfoto). Foto: DESY, Jan Kaiser
The team experimented with 14 different LLMs and used them to control ARES, an experimental electron accelerator at DESY. Their task was to adjust five magnets in such a way that the electron beam from ARES was focused and steered in the best possible way. Instead of ‘coding’ the requirements, however, the scientists described the problem to the LLMs using natural language prompts. The LLMs then suggested how to set the five tuning parameters with the utmost precision, explaining what each value would achieve.
“We were totally surprised at how well some of the LLMs were able to solve the problem,” says Kaiser. “What’s even more fascinating, though, is that they don’t even need to know that they are operating a particle accelerator. So in theory, LLMs can solve any type of optimisation problem, which means that our approach is relevant far beyond the field of accelerator physics.”
Of course, the researchers also encountered certain obstacles during their proof-of-concept investigations. “Just like with human operators, you have to ask the right LLM the right question to get a good solution,” says Annika Eichler (DESY and Hamburg University of Technology), whose research group is responsible for the intelligent control of accelerator systems using AI. The computational power and thus the environmental impact of solving such problem is currently substantial. Furthermore, the systems are still lagging behind other specialised methods. For the time being, LLMs would probably be better serving as advisory ‘co-pilots’ in accelerator control rooms.
“Nevertheless, our work is an important first step towards a future in which complex and highly relevant scientific facilities can be operated simply by asking for the desired result using natural language,” says Eichler.
Reference
Large language models for human-machine collaborative particle accelerator tuning through natural language. Jan Kaiser, Anne Lauscher and Annika Eichler. Science Advances. 2025. DOI: 10.1126/sciadv.adr4173